The history of statistics and soccer is a short one. American sports fans started counting things as soon as there were things to count. The static nature of baseball lends itself to box scores, and then baseball’s box scores led to everybody else following suit.
Soccer didn’t develop that way. The fluid nature of the game meant that for most of its existence nobody officially recorded anything other than goals. It wasn’t until well into the 21st Century that intrepid private data collectors began watching the game with an eye toward recording everything that went on.
The wealth of new data hasn’t always been used well. Coverage of soccer is now stuffed with the kinds of statistics that might mean nothing at all, a proliferation of RBI but precious few WARs, so to speak.
Most of the best statistical work is going on quietly, behind the scenes, where sharp minds both inside and outside the game sort through all the data and build the important tools that actually hold predictive power.
Here are some of the statistical do's and don’ts of soccer: The basic stats that are useful, the ones that aren’t and some of the more complicated stats that the most serious sharps are fluent in.
The Basics
Per 90s
It’s a simple modifier but an important one when it comes to player stats. Normalize stats, even basic ones such as goals and assists, for the amount of minutes a player is actually playing.
Too often players' stats are counted only as raw numbers, or at most on a per-game basis. But, a player's numbers can look really different if that player is coming off the bench late in the game or starting on a regular basis.
This is particularly important on the attacking end of the field. It’s easy to miss players who are really good at generating shots and goals for themselves and their teammates as substitutes.
Their raw numbers, or their per-appearance numbers will make them look like mediocre attacking players when in fact they are being incredibly productive. On the flip side, ironmen who are great at playing every game — but don’t necessarily put up the flashy stats — can get overvalued by season’s end without adjusting for playing time.
Shots
It sounds obvious, but the number of shots a team takes and concedes turns out to be really important, and a fairly predictive measure of future success.
Building on the work of hockey, early soccer analysts used tools such as Total Shot Ratio and PDO as tools for predicting future success based on past shots, and it works quite well.
The challenge of soccer analytics is that there really are very few goals, so analyzing a team’s strength simply based on goals scored and conceded simply doesn’t provide enough information to make accurate predictions.
At least, not if you don’t want error bars so gigantic that they make the predictions so vague that they essentially become useless. Using shots means more data, and that data is surprisingly predictive.
Shots aren’t perfect. There are, of course, good shots and bad shots. And some teams are better at taking more of the former, and others rely heavily on the latter.
But, for a basic statistic, simply looking at the difference between the number of shots a team takes and the number of shots a team concedes does a pretty good job as the basis for modeling a team’s attacking and defending strengths.
Shots are similarly useful when evaluating attacking players. Almost all great attacking players take a lot of shots.
>> Sign up for The Action Network's daily newsletter to get the smartest conversation delivered into your inbox each morning.
The idea of the player who is relatively conservative about firing away but is a deadeye when he hits the ball is largely a myth. Part of what makes great soccer scorers great is that they are able to get their shots off.
Similarly to evaluating teams, it can be tricky to account for players who take a lot of bad shots. Players who pad their numbers by taking shots from exceedingly long range — or off set pieces regardless of whether it’s a good idea — can trick naïve shot-based metrics.
But, after accounting for that (one useful way is to also look at shots within the 18-yard box as well as total shots), looking for players generating a lot of shots is a good way to find guys who are going to score a lot of goals.
The Common Mistakes
Shooting Percentage:
Players who score on a high percentage of the shots they take are not necessarily good shooters. A player’s shooting percentage is defined mostly by two components — first, the kinds of shots they take, and second, dumb luck. It is, of course, silly to say that some players aren’t better at kicking a ball more accurately than others.
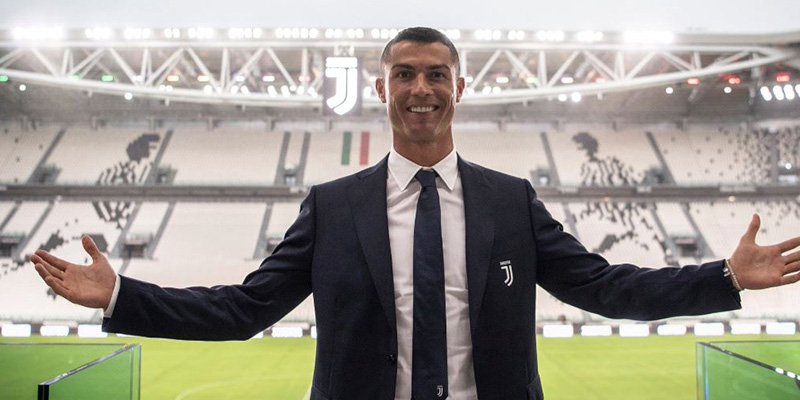
They are. But, over the course of a game or a season, or five seasons, the amount that those skill differences matter pales in comparison to both the amount of the kinds of shots they take matters, and blind dumb variance. Your first reaction to a player with a sky-high shooting percentage should be to expect that player to come back to earth, not to predict he's the next Lionel Messi.
Possession
Possession statistics are the kings of the correlation-doesn’t-mean-causation prom. Good teams tend to have high possession statistics.
That is because good teams tend to have good players, and good players tend to be good at doing things such as passing the ball accurately to other good players, or taking the ball from bad players on the other team. But, playing a high-possession style does not, in and of itself, make a team good.
There are plenty of club teams, such as Chelsea or Atletico Madrid that have chosen to play a style that focuses on defending without the ball and counterattacking effectively. Those teams maximize their personnel and tactical choices to effectively execute that style, and they are perfectly capable of staying at the top of European soccer by doing that.
The fact that they have a lower possession style doesn’t inhibit them from performing well on either basic or more advanced metrics, and it doesn’t inhibit them from winning.
This is particularly important in international tournaments where teams are formed by nationality and not by professional planning. Collections of talent are unavoidably awkward fits, as opposed to being cohesive units.
The top teams in the world such as Germany, Spain or Brazil all fit together and are happy to be possession machines. But for many others, the talent pool leads to adopting defensive strategies that downplay possession in favor of conservative positioning. Trying to judge middle-tier World Cup squads by their possession percentage is a recipe for disaster.
The Advanced Stats
Expected Goals
Expected goals remains the gold standard for predicting future performance. Conceptually the idea is simple. Instead of counting shots, give each shot a value reflecting the likelihood it will be a goal.
Instead of looking at goals, or shots, look at expected goals taken and conceded. That does a better job of predicting future results. Building team ratings both in attack and defense based on some form of xG is definitely a best practice.
The devil is in the details. How exactly to best evaluate a shot’s chances of being scored isn’t a simple question. Nor is having the data to do it accurately. Some aspects are easy to isolate.
Where on the field is the shot from, both distance and angle? What part of the body is used to take the shot? Headers, for example, result in fewer goals than kicked shots do. Was the shot from open play or from a set piece?
Other things are harder to quantify. Defensive positioning is the biggest hurdle. Most, although not all, soccer data focuses on on-ball events. It is blind to how defenses are positioned or where the keeper is.
Smart xG models work around this by using factors such as the speed of attack, the kind of pass that led to a given shot and whether a player dribbled past a player right before a shot to glean information about the surrounding circumstances.
Not all xG models are created equally, and the best ones find clever ways to incorporate all the data they can get their hands on.
From a pure predictive modeling perspective nothing much tops xG.
Non-Shot Based xG:
One particular hole in xG models is that they’re built entirely on shots. They’re entirely blind to possessions that end with other kinds of actions, such as incomplete passes or turnovers or any of the other million things that happen during the run of play.
This isn’t a huge problem. xG works well despite those actions. But, it is an area where other modeling solutions can bring more information to the party.
Some xG models try to augment the information they’re built on by adding in other factors — ones that are designed to help determine whether a team might be better or worse at turning possession itself into shots that result in goals.
The idea here is to quickly identify teams that are putting up higher or lower xG numbers than the rest of their statistics might indicate they should and predict more accurately how those teams will perform.
This mostly involves modeling passing and the volume and type of passing that teams are doing in the final third of the field, and then predicting how often that passing should result in shots as opposed to how often it has been resulting in shots.
That then informs the modeling of the values of the shots the team has taken. It’s an added layer of complexity.
An xG model predicts how many goals a team “should” have scored from the shots it has taken, while a non-shot xG model is predicting how many goals a team should have scored from the shots it has taken and the pass it has made.
xGChain
The idea behind xGChain is to take the concept of xG and extend it backward to players who were involved in creating the shot that xG measures. One of the major shortcomings of xG as a statistic is that it’s useful only on a player level for the guys who take shots.
Midfielders who spend their time orchestrating play and contributing to creating attacks aren’t captured by what it measures.
A striker who benefits from a midfield maestro who continually puts him in great positions to score gets credit, while the maestro remains, as far as xG is concerned, a black box.
So, what xGChain does is take xG and move it backward along the chain of possession. Each shot becomes the summation of the series of events that led up to it.
That way the players who win the ball back and play the passes that ultimately, several actions later, lead to a shot get credit.
It’s less about predicting team performance and more about isolating who, exactly, is responsible for that performance. It’s obviously useful for club teams evaluating which players to buy and sell.
In the international game context, it's a tool that helps determine which lineups might play well together and whether particular injuries or suspensions might have an outsized impact on a team’s performance in ways that more basic passing stats wouldn’t necessarily pick up.